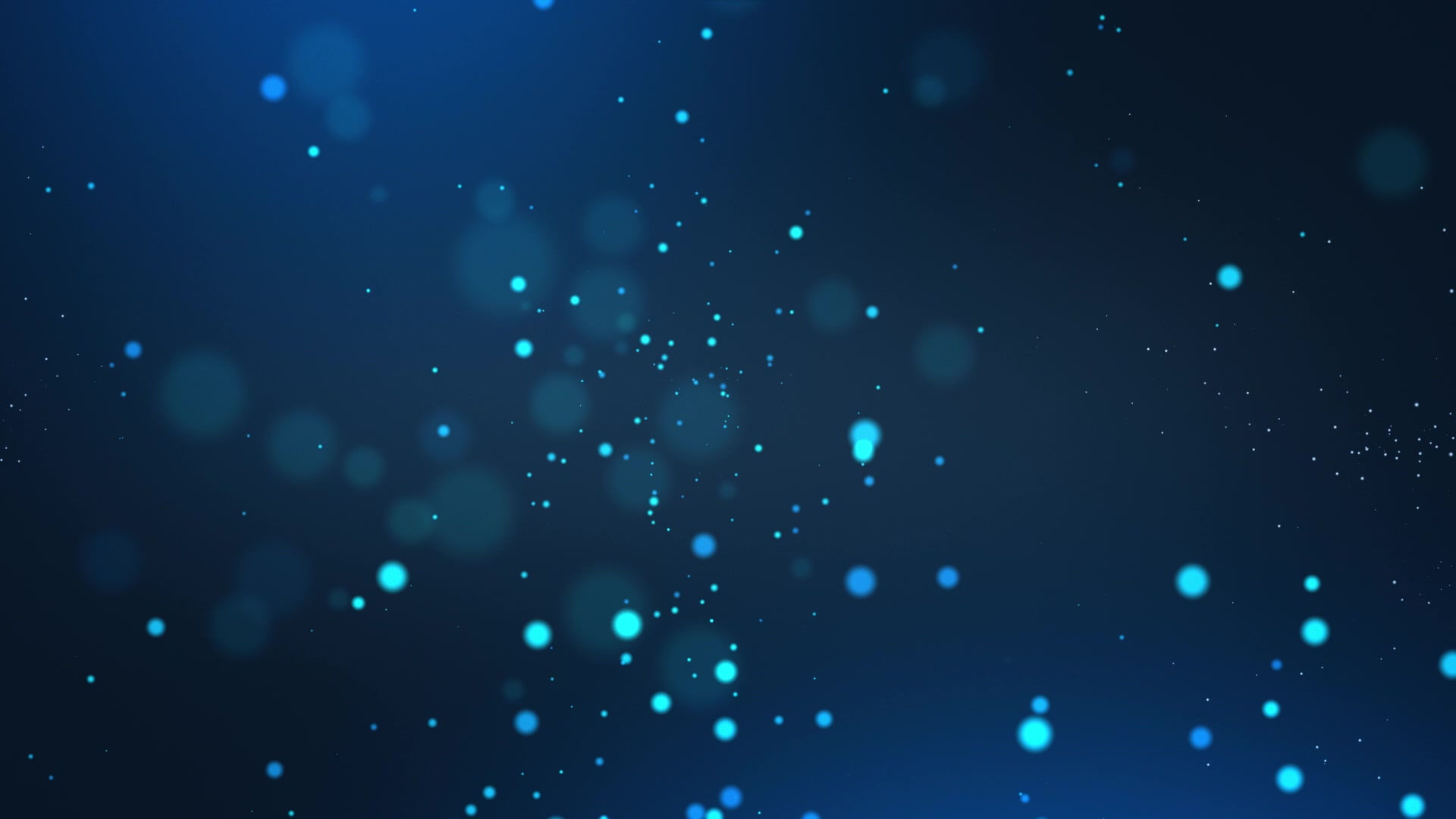
Variable Selection
Outlier Treatment
Variable which are not important we simple remove for building model in the dataset. Here we remove following the variable:-
-
Item Identifier.
-
Outlet Identifier.
-
Outlet Establishment year.
This two variable has no impact in our model building part. So we removed and make our model more reliable.
And other side we are create one more variable for our model building part. This variable are helping better to handle data. We have variable Outlet Establishment year but this variable are not much impactful for the analysis. So we create a new variable “Age of Shops” this variable. This is easiest for analysis. Each Outlet establishment Year are subtracting for 2013. Because 2103 year is our base year. All the data are collect end of 2013.
From the given data we need to find outlier. So an outlier is an observation point that is distant from other observations. An outlier may be due to variability in the measurement or it may indicate experimental error; the latter are sometimes excluded from the data set. So in my data set Item visibility are having some outlier. Here outlier detect through box plot.
So in this case I’m treat outlier through square root transform. Also item visibility having lots of ‘0’ values but there not significant atoll so we replace ‘0’ visibility with median value of visibility.
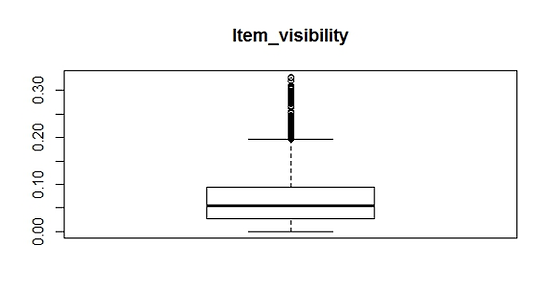